T7 - Calibration#
An interactive version of this notebook is available on Google Colab or Binder.
Disease models typically require contextualization to a relevant setting of interest prior to addressing “what-if” scenario questions. The process of tuning model input parameters so that model outputs match observed data is known as calibration. There are many approaches to model calibration, ranging from manual tuning to fully Bayesian methods.
For many applications, we have found that an optimization-based approach is sufficient. Such methods avoid the tedious process of manual tuning and are less computationally expensive than fully Bayesian methods. One such optimization-based approach is the Optuna library, which is a Bayesian hyperparameter optimization framework. Optuna is designed for tuning hyperparameters of machine learning models, but it can also be used to calibrate disease models.
Calibration libraries often treat the disease model as a black box, where the input parameters are the “hyperparameters” to be tuned. The calibration process is often iterative and requires a combination of expert knowledge and computational tools. The optimization algorithm iteratively chooses new parameter values to evaluate, and the model is run with these values to generate outputs. The outputs are compared to observed data, and a loss function is calculated to quantify the difference between the model outputs and the observed data. The optimization algorithm then uses this loss function to update its search strategy and choose new parameter values to evaluate. This process continues until the algorithm converges to a set of parameter values that minimize the loss function.
While many optimization algorithms are available, Starsim has a built-in interface to the Optuna library, which we will demonstrate in this tutorial. We will use a simple Susceptible-Infected-Recovered (SIR) model as an example. We will tune three input parameters, the infectivity parameter, beta
, the initial prevalence parameter, init_prev
, and the Poisson-distributed degree distribution parameter, n_contacts
. We will calibrate the model using a beta-binomial likelihood function so
as to match prevalence at three distinct time points.
We begin with a few imports and default settings:
[1]:
#%% Imports and settings
import sciris as sc
import starsim as ss
import pandas as pd
n_agents = 2e3
debug = False # If true, will run in serial
The calibration class will require a base Sim
object. This sim
will later be modified according to parameters selected by the optimization engine. The following function creates the base Sim
object.
[2]:
def make_sim():
""" Helper function to create the base simulation object """
sir = ss.SIR(
beta = ss.beta(0.075),
init_prev = ss.bernoulli(0.02),
)
random = ss.RandomNet(n_contacts=ss.poisson(4))
sim = ss.Sim(
n_agents = n_agents,
start = sc.date('1990-01-01'),
dur = 40,
dt = 1,
unit = 'day',
diseases = sir,
networks = random,
verbose = 0,
)
# Remember to return the sim object
return sim
Now let’s define the calibration parameters. These are the inputs that Optuna will be able to modify. Here, we define three such parameters, beta
, init_prev
, and n_contacts
.
Each parameter entry should have range defined by low
and high
as well as a guess
values. The guess
value is not used by Optuna, rather only for a check after calibration completes to see if the new parameters are better than the guess
values.
You’ll notice there are a few other parameters that can be specified. For example, the data type of the parameter appears in suggest_type
. Possible values are listed in the Optuna documentation, and include suggest_float for float values and
suggest_int for integer types.
To make things easier for the search algorithm, it’s helpful to indicate how outputs are expected to change with inputs. For example, increasing beta
from 0.01 to 0.02 should double disease transmission, but increasing from 0.11 to 0.12 will have a small effect. Thus, we indicate that this parameter should be calibrated with log=True
.
[3]:
# Define the calibration parameters
calib_pars = dict(
beta = dict(low=0.01, high=0.30, guess=0.15, suggest_type='suggest_float', log=True), # Note the log scale
init_prev = dict(low=0.01, high=0.05, guess=0.15), # Default type is suggest_float, no need to re-specify
n_contacts = dict(low=2, high=10, guess=3, suggest_type='suggest_int'), # Suggest int just for this demo
)
The optimization engine iteratively chooses input parameters to simulate. Those parameters are passed into the following build_sim
function as a dictionary of calib_pars
along with the base sim
and any other key word arguments. The calib_pars
will be as above, but importantly will have an additional key named value
containing the value selected by Optuna.
When modifying a sim
, it is important to realize that the simulation has not been initialized yet. Nonetheless, the configuration is available for modification at sim.pars
, as demonstrated in the function below for the SIR example.
[4]:
def build_sim(sim, calib_pars, **kwargs):
""" Modify the base simulation by applying calib_pars """
sir = sim.pars.diseases # There is only one disease in this simulation and it is a SIR
net = sim.pars.networks # There is only one network in this simulation and it is a RandomNet
for k, pars in calib_pars.items(): # Loop over the calibration parameters
if k == 'rand_seed':
sim.pars.rand_seed = v
continue
# Each item in calib_pars is a dictionary with keys like 'low', 'high',
# 'guess', 'suggest_type', and importantly 'value'. The 'value' key is
# the one we want to use as that's the one selected by the algorithm
v = pars['value']
if k == 'beta':
sir.pars.beta = ss.beta(v)
elif k == 'init_prev':
sir.pars.init_prev = ss.bernoulli(v)
elif k == 'n_contacts':
net.pars.n_contacts = ss.poisson(v)
else:
raise NotImplementedError(f'Parameter {k} not recognized')
return sim
The Starsim framework has been integrated with the Optuna hyperparameter optimization algorithm to facilitate calibration through the Calibration
class. Recall that an optimization-based approach to calibration minimizes a function of the input parameters. This function is key to achieving an acceptable calibration.
There are two ways to describe the goodness-of-fit function for the Calibration
. The first method is to directly provide a function that the algorithm will call. The eval_fn
will be passed each completed sim
after running, and is expected to return a float representing the goodness of fit (higher is better). Data can be passed into the eval_fn
via eval_kwargs
.
As an alternative to directly specifying the evaluation function, you can use CalibComponent
s. Each component includes real data, for example from a survey, that is compared against simulation data from the model. Several components and be used at the same time, for example one for disease prevalence and another for treatment coverage. Each component computes a likelihood of the data given the input parameters, as assess via simulation. Components are combined assuming independence.
When defining a CalibComponent
, we give it a name
and pass in expected
(the real data to be calibrated to). The required data fields depend on the likelihood function. Importantly, the functional form of the negative log likelihood, or nll, is defined by the nll_fn
. The value for nll_fn
can be 'beta'
, 'gamma'
, or a negative log likelihood function of your own creation. If designing your own function for nll_fn
, it should take two arguments: expected
and
actual
. For a beta binomial, the data must define n
and x
, where n
is the number of individuals who were sampled and x
is the number that were found, e.g. identified as positive.
Output from the simulation is obtained via a function. The function takes a completed sim
object as input and returns a dictionary with fields as required for the evaluation function of your choice. In the example below, we use an in-line lambda function to extract n
and x
from the simulation, as required by the Beta binomial component.
Each component has a weight
. The final goodness of fit is a weighted sum of negative log likelihoods.
Finally, the conform
argument describes how the simulation output is adjusted to align with the real data. For example, if the real data is a prevalence measurement, choosing 'prevalent'
will interpolate the simulation output at the time points of the real data. Choosing 'incident'
, the simulation output will be aggregated between time points of the real data.
[5]:
infectious = ss.CalibComponent(
name = 'Infectious',
# For this example, the "expected" comes from a simulation with pars
# beta=0.075, init_prev=0.02, n_contacts=4
expected = pd.DataFrame({
'n': [200, 197, 195], # Number of individuals sampled
'x': [30, 30, 10], # Number of individuals found to be infectious
}, index=pd.Index([ss.date(d) for d in ['1990-01-12', '1990-01-25', '1990-02-02']], name='t')), # On these dates
extract_fn = lambda sim: pd.DataFrame({
'n': sim.results.n_alive, # Number of individuals sampled
'x': sim.results.sir.n_infected, # Number of individuals found to be infectious
}, index=pd.Index(sim.results.timevec, name='t')), # Index is time
conform = 'prevalent',
nll_fn = 'beta',
weight = 1, # Not required if only one component
)
Finally, we can bring all the pieces together. We make a single base simulation and create an instance of a Starsim Calibration object. This object requires a few arguments, like the calib_pars
and sim
. We also pass in the function that modifies the base sim
, here our build_sim
function. No additional build_kw
are required in this example.
We also pass in a list of components
. Instead of using this “component-based” system, a user could simply provide an eval_fn
, which takes in a completed sim an any eval_kwargs
and returns a “goodness of fit” score to be maximized.
We can also specify the total number of trial to run, the number of parallel works, and a few other parameters.
[6]:
sc.heading('Beginning calibration')
# Make the sim and data
sim = make_sim()
# Make the calibration
calib = ss.Calibration(
calib_pars = calib_pars,
sim = sim,
build_fn = build_sim, # Use default builder, Calibration.translate_pars
build_kw = None,
components = [infectious],
total_trials = 100,
n_workers = None, # None indicates to use all available CPUs
die = True,
debug = debug,
)
# Perform the calibration
sc.printcyan('\nPeforming calibration...')
calib.calibrate();
—————————————————————
Beginning calibration
—————————————————————
Peforming calibration...
Removed existing calibration file starsim_calibration.db
sqlite:///starsim_calibration.db
[I 2024-11-18 15:45:43,530] A new study created in RDB with name: starsim_calibration
[I 2024-11-18 15:45:44,965] Trial 1 finished with value: 154.74468717333798 and parameters: {'beta': 0.019491203730843808, 'init_prev': 0.01054601270105202, 'n_contacts': 5, 'rand_seed': 718373}. Best is trial 0 with value: 15.980858702644355.
[I 2024-11-18 15:45:44,978] Trial 0 finished with value: 15.980858702644355 and parameters: {'beta': 0.04892094210179355, 'init_prev': 0.020119785912875887, 'n_contacts': 7, 'rand_seed': 748746}. Best is trial 0 with value: 15.980858702644355.
[I 2024-11-18 15:45:45,379] Trial 2 finished with value: 113.77535549216077 and parameters: {'beta': 0.22856885717998712, 'init_prev': 0.04938909252740796, 'n_contacts': 8, 'rand_seed': 333928}. Best is trial 0 with value: 15.980858702644355.
[I 2024-11-18 15:45:45,411] Trial 3 finished with value: 146.88160556520666 and parameters: {'beta': 0.012697218127524177, 'init_prev': 0.01112215866505724, 'n_contacts': 10, 'rand_seed': 498194}. Best is trial 0 with value: 15.980858702644355.
[I 2024-11-18 15:45:45,796] Trial 4 finished with value: 15.893816546413973 and parameters: {'beta': 0.0781191571037374, 'init_prev': 0.04206124846222018, 'n_contacts': 4, 'rand_seed': 497387}. Best is trial 4 with value: 15.893816546413973.
[I 2024-11-18 15:45:45,824] Trial 5 finished with value: 138.30823678144384 and parameters: {'beta': 0.2713976098751463, 'init_prev': 0.03661138889687137, 'n_contacts': 3, 'rand_seed': 886038}. Best is trial 4 with value: 15.893816546413973.
[I 2024-11-18 15:45:46,233] Trial 6 finished with value: 101.98306428803221 and parameters: {'beta': 0.16622677008657166, 'init_prev': 0.023103266187331398, 'n_contacts': 10, 'rand_seed': 255848}. Best is trial 4 with value: 15.893816546413973.
[I 2024-11-18 15:45:46,285] Trial 7 finished with value: 8.083722405425533 and parameters: {'beta': 0.0388554073114571, 'init_prev': 0.03159220090689648, 'n_contacts': 8, 'rand_seed': 894673}. Best is trial 7 with value: 8.083722405425533.
[I 2024-11-18 15:45:46,659] Trial 8 finished with value: 117.21373623754403 and parameters: {'beta': 0.20774295565646272, 'init_prev': 0.03903543706977345, 'n_contacts': 10, 'rand_seed': 255753}. Best is trial 7 with value: 8.083722405425533.
[I 2024-11-18 15:45:46,718] Trial 9 finished with value: 101.0013439458387 and parameters: {'beta': 0.022393073929320502, 'init_prev': 0.04740276477404884, 'n_contacts': 5, 'rand_seed': 239984}. Best is trial 7 with value: 8.083722405425533.
[I 2024-11-18 15:45:47,028] Trial 10 finished with value: 167.13709732073653 and parameters: {'beta': 0.024373096962236714, 'init_prev': 0.046214735204025234, 'n_contacts': 2, 'rand_seed': 950612}. Best is trial 7 with value: 8.083722405425533.
[I 2024-11-18 15:45:47,204] Trial 11 finished with value: 16.006833628419145 and parameters: {'beta': 0.044595971141575756, 'init_prev': 0.029515307336862504, 'n_contacts': 8, 'rand_seed': 3568}. Best is trial 7 with value: 8.083722405425533.
[I 2024-11-18 15:45:47,504] Trial 12 finished with value: 7.910936974549941 and parameters: {'beta': 0.07352780536205664, 'init_prev': 0.032413746410370356, 'n_contacts': 4, 'rand_seed': 514334}. Best is trial 12 with value: 7.910936974549941.
[I 2024-11-18 15:45:47,735] Trial 13 finished with value: 26.38781967333739 and parameters: {'beta': 0.09128798730890926, 'init_prev': 0.03762231428814388, 'n_contacts': 4, 'rand_seed': 559562}. Best is trial 12 with value: 7.910936974549941.
[I 2024-11-18 15:45:48,221] Trial 14 finished with value: 145.59512706882083 and parameters: {'beta': 0.08867951945516166, 'init_prev': 0.03154583217260131, 'n_contacts': 6, 'rand_seed': 684664}. Best is trial 12 with value: 7.910936974549941.
[I 2024-11-18 15:45:48,365] Trial 15 finished with value: 152.6652957120184 and parameters: {'beta': 0.1125215604980274, 'init_prev': 0.030913443125467805, 'n_contacts': 7, 'rand_seed': 788866}. Best is trial 12 with value: 7.910936974549941.
[I 2024-11-18 15:45:48,693] Trial 16 finished with value: 8.969493607989307 and parameters: {'beta': 0.03455352909043222, 'init_prev': 0.029260666473231216, 'n_contacts': 8, 'rand_seed': 996547}. Best is trial 12 with value: 7.910936974549941.
[I 2024-11-18 15:45:48,838] Trial 17 finished with value: 9.881846707547766 and parameters: {'beta': 0.03488348260273924, 'init_prev': 0.023898874933101687, 'n_contacts': 8, 'rand_seed': 989802}. Best is trial 12 with value: 7.910936974549941.
[I 2024-11-18 15:45:49,149] Trial 18 finished with value: 116.90626643075734 and parameters: {'beta': 0.06436895384616079, 'init_prev': 0.023198369691759008, 'n_contacts': 2, 'rand_seed': 604295}. Best is trial 12 with value: 7.910936974549941.
[I 2024-11-18 15:45:49,300] Trial 19 finished with value: 142.54432303383214 and parameters: {'beta': 0.06170915830253712, 'init_prev': 0.018548570400842186, 'n_contacts': 2, 'rand_seed': 596260}. Best is trial 12 with value: 7.910936974549941.
[I 2024-11-18 15:45:49,820] Trial 20 finished with value: 195.40027291482215 and parameters: {'beta': 0.1350893485234926, 'init_prev': 0.01718825596886564, 'n_contacts': 6, 'rand_seed': 401422}. Best is trial 12 with value: 7.910936974549941.
[I 2024-11-18 15:45:49,948] Trial 21 finished with value: 141.1310761215816 and parameters: {'beta': 0.13580463972604376, 'init_prev': 0.034305287339592386, 'n_contacts': 6, 'rand_seed': 422249}. Best is trial 12 with value: 7.910936974549941.
[I 2024-11-18 15:45:50,322] Trial 22 finished with value: 10.660142746960219 and parameters: {'beta': 0.03515972207525765, 'init_prev': 0.033331018269778426, 'n_contacts': 9, 'rand_seed': 860029}. Best is trial 12 with value: 7.910936974549941.
[I 2024-11-18 15:45:50,442] Trial 23 finished with value: 7.745931318336034 and parameters: {'beta': 0.03362190608800137, 'init_prev': 0.027885507852357612, 'n_contacts': 9, 'rand_seed': 856410}. Best is trial 23 with value: 7.745931318336034.
[I 2024-11-18 15:45:51,178] Trial 25 finished with value: 82.21696849347313 and parameters: {'beta': 0.01741950427515872, 'init_prev': 0.026861980735413037, 'n_contacts': 9, 'rand_seed': 839399}. Best is trial 23 with value: 7.745931318336034.
[I 2024-11-18 15:45:51,273] Trial 24 finished with value: 7.4876053262421465 and parameters: {'beta': 0.03299869908695853, 'init_prev': 0.0273236673469651, 'n_contacts': 9, 'rand_seed': 856084}. Best is trial 24 with value: 7.4876053262421465.
[I 2024-11-18 15:45:51,965] Trial 26 finished with value: 20.638330536063904 and parameters: {'beta': 0.04253010820328066, 'init_prev': 0.02719769337580837, 'n_contacts': 9, 'rand_seed': 89943}. Best is trial 24 with value: 7.4876053262421465.
[I 2024-11-18 15:45:52,029] Trial 27 finished with value: 13.931178918863907 and parameters: {'beta': 0.02728393349110877, 'init_prev': 0.025809341510766266, 'n_contacts': 9, 'rand_seed': 83877}. Best is trial 24 with value: 7.4876053262421465.
[I 2024-11-18 15:45:52,676] Trial 28 finished with value: 131.90073698725678 and parameters: {'beta': 0.013573983516972348, 'init_prev': 0.040806742706758894, 'n_contacts': 7, 'rand_seed': 651514}. Best is trial 24 with value: 7.4876053262421465.
[I 2024-11-18 15:45:52,717] Trial 29 finished with value: 162.69901960867605 and parameters: {'beta': 0.010586951770277957, 'init_prev': 0.01609112387914806, 'n_contacts': 7, 'rand_seed': 675639}. Best is trial 24 with value: 7.4876053262421465.
[I 2024-11-18 15:45:53,165] Trial 30 finished with value: 11.704591123940872 and parameters: {'beta': 0.0530628354297839, 'init_prev': 0.020500428143174473, 'n_contacts': 5, 'rand_seed': 802469}. Best is trial 24 with value: 7.4876053262421465.
[I 2024-11-18 15:45:53,214] Trial 31 finished with value: 10.906209496638212 and parameters: {'beta': 0.056340730331552805, 'init_prev': 0.020289712981291754, 'n_contacts': 5, 'rand_seed': 786383}. Best is trial 24 with value: 7.4876053262421465.
[I 2024-11-18 15:45:54,083] Trial 33 finished with value: 8.055721660258087 and parameters: {'beta': 0.03227167204321693, 'init_prev': 0.03261826772048466, 'n_contacts': 9, 'rand_seed': 925371}. Best is trial 24 with value: 7.4876053262421465.
[I 2024-11-18 15:45:54,134] Trial 32 finished with value: 7.8882042054453905 and parameters: {'beta': 0.029897456958550325, 'init_prev': 0.033997365932046136, 'n_contacts': 9, 'rand_seed': 917877}. Best is trial 24 with value: 7.4876053262421465.
[I 2024-11-18 15:45:54,693] Trial 34 finished with value: 28.250985079301927 and parameters: {'beta': 0.01983573326361735, 'init_prev': 0.03611273773305575, 'n_contacts': 10, 'rand_seed': 730021}. Best is trial 24 with value: 7.4876053262421465.
[I 2024-11-18 15:45:54,926] Trial 35 finished with value: 37.30420459278935 and parameters: {'beta': 0.018560655525111658, 'init_prev': 0.03608361906456892, 'n_contacts': 10, 'rand_seed': 746022}. Best is trial 24 with value: 7.4876053262421465.
[I 2024-11-18 15:45:55,219] Trial 36 finished with value: 122.39866550486727 and parameters: {'beta': 0.027438204756350858, 'init_prev': 0.028338472823034387, 'n_contacts': 4, 'rand_seed': 748420}. Best is trial 24 with value: 7.4876053262421465.
[I 2024-11-18 15:45:55,382] Trial 37 finished with value: 121.83497223931477 and parameters: {'beta': 0.028579693265723682, 'init_prev': 0.0283061623766216, 'n_contacts': 4, 'rand_seed': 832593}. Best is trial 24 with value: 7.4876053262421465.
[I 2024-11-18 15:45:56,104] Trial 38 finished with value: 127.78035821504159 and parameters: {'beta': 0.06979522357047054, 'init_prev': 0.04362492485249174, 'n_contacts': 7, 'rand_seed': 842333}. Best is trial 24 with value: 7.4876053262421465.
[I 2024-11-18 15:45:56,465] Trial 39 finished with value: 26.752967538334588 and parameters: {'beta': 0.07081946402820172, 'init_prev': 0.025283604391157108, 'n_contacts': 3, 'rand_seed': 934584}. Best is trial 24 with value: 7.4876053262421465.
[I 2024-11-18 15:45:57,009] Trial 40 finished with value: 15.521521816261838 and parameters: {'beta': 0.045541805017461245, 'init_prev': 0.025293131832945914, 'n_contacts': 8, 'rand_seed': 926071}. Best is trial 24 with value: 7.4876053262421465.
[I 2024-11-18 15:45:57,158] Trial 41 finished with value: 45.90869881112826 and parameters: {'beta': 0.04854941897250185, 'init_prev': 0.013488443491240563, 'n_contacts': 9, 'rand_seed': 459644}. Best is trial 24 with value: 7.4876053262421465.
[I 2024-11-18 15:45:57,573] Trial 42 finished with value: 7.827706028795319 and parameters: {'beta': 0.030879125658603422, 'init_prev': 0.033649204256261514, 'n_contacts': 9, 'rand_seed': 943454}. Best is trial 24 with value: 7.4876053262421465.
[I 2024-11-18 15:45:58,236] Trial 43 finished with value: 8.164985957065028 and parameters: {'beta': 0.03241585863699491, 'init_prev': 0.034639610917373546, 'n_contacts': 9, 'rand_seed': 910120}. Best is trial 24 with value: 7.4876053262421465.
[I 2024-11-18 15:45:58,371] Trial 44 finished with value: 67.41735979311738 and parameters: {'beta': 0.015476742238041482, 'init_prev': 0.034159802667364444, 'n_contacts': 10, 'rand_seed': 908810}. Best is trial 24 with value: 7.4876053262421465.
[I 2024-11-18 15:45:58,767] Trial 45 finished with value: 17.184628220332115 and parameters: {'beta': 0.023442549485332725, 'init_prev': 0.0384392278879358, 'n_contacts': 10, 'rand_seed': 877016}. Best is trial 24 with value: 7.4876053262421465.
[I 2024-11-18 15:45:58,901] Trial 46 finished with value: 19.39283513487203 and parameters: {'beta': 0.023091385039949813, 'init_prev': 0.03893135979666424, 'n_contacts': 10, 'rand_seed': 975476}. Best is trial 24 with value: 7.4876053262421465.
[I 2024-11-18 15:45:59,299] Trial 47 finished with value: 60.579286248094604 and parameters: {'beta': 0.02205540239945062, 'init_prev': 0.030491151609991437, 'n_contacts': 8, 'rand_seed': 336962}. Best is trial 24 with value: 7.4876053262421465.
[I 2024-11-18 15:45:59,424] Trial 48 finished with value: 10.171996450363395 and parameters: {'beta': 0.03994956089664571, 'init_prev': 0.03245382957019944, 'n_contacts': 8, 'rand_seed': 794503}. Best is trial 24 with value: 7.4876053262421465.
[I 2024-11-18 15:45:59,829] Trial 49 finished with value: 8.04642903290619 and parameters: {'beta': 0.03727480097329536, 'init_prev': 0.032457596882781145, 'n_contacts': 8, 'rand_seed': 536249}. Best is trial 24 with value: 7.4876053262421465.
[I 2024-11-18 15:45:59,903] Trial 50 finished with value: 17.156371712485225 and parameters: {'beta': 0.0934687860320593, 'init_prev': 0.041042881711329034, 'n_contacts': 3, 'rand_seed': 689114}. Best is trial 24 with value: 7.4876053262421465.
[I 2024-11-18 15:46:00,456] Trial 51 finished with value: 15.033986831375614 and parameters: {'beta': 0.029113141906577416, 'init_prev': 0.04150393257652148, 'n_contacts': 9, 'rand_seed': 965284}. Best is trial 24 with value: 7.4876053262421465.
[I 2024-11-18 15:46:00,696] Trial 52 finished with value: 17.902465172270922 and parameters: {'beta': 0.03873712956159845, 'init_prev': 0.030450717339511737, 'n_contacts': 9, 'rand_seed': 510268}. Best is trial 24 with value: 7.4876053262421465.
[I 2024-11-18 15:46:01,423] Trial 53 finished with value: 8.462873114877539 and parameters: {'beta': 0.03983981971033295, 'init_prev': 0.0300566758063839, 'n_contacts': 8, 'rand_seed': 510305}. Best is trial 24 with value: 7.4876053262421465.
[I 2024-11-18 15:46:01,617] Trial 54 finished with value: 53.899274775888216 and parameters: {'beta': 0.05422374676712681, 'init_prev': 0.035748222707034406, 'n_contacts': 8, 'rand_seed': 545960}. Best is trial 24 with value: 7.4876053262421465.
[I 2024-11-18 15:46:02,361] Trial 55 finished with value: 63.2285833053927 and parameters: {'beta': 0.05483140005782871, 'init_prev': 0.03520644816493804, 'n_contacts': 8, 'rand_seed': 551658}. Best is trial 24 with value: 7.4876053262421465.
[I 2024-11-18 15:46:02,629] Trial 56 finished with value: 10.526111429321418 and parameters: {'beta': 0.025517731049574686, 'init_prev': 0.028683362138683714, 'n_contacts': 10, 'rand_seed': 621414}. Best is trial 24 with value: 7.4876053262421465.
[I 2024-11-18 15:46:03,191] Trial 57 finished with value: 13.322795378645196 and parameters: {'beta': 0.026189504774735663, 'init_prev': 0.032294688542589184, 'n_contacts': 9, 'rand_seed': 377222}. Best is trial 24 with value: 7.4876053262421465.
[I 2024-11-18 15:46:03,328] Trial 58 finished with value: 160.41268298855516 and parameters: {'beta': 0.08035649151889546, 'init_prev': 0.03232659371949306, 'n_contacts': 9, 'rand_seed': 355327}. Best is trial 24 with value: 7.4876053262421465.
[I 2024-11-18 15:46:03,827] Trial 59 finished with value: 26.672051646097998 and parameters: {'beta': 0.03091813502331794, 'init_prev': 0.021643916677641817, 'n_contacts': 7, 'rand_seed': 288520}. Best is trial 24 with value: 7.4876053262421465.
[I 2024-11-18 15:46:04,155] Trial 60 finished with value: 16.727160301691356 and parameters: {'beta': 0.031033050131487494, 'init_prev': 0.0374589240214719, 'n_contacts': 7, 'rand_seed': 274596}. Best is trial 24 with value: 7.4876053262421465.
[I 2024-11-18 15:46:04,904] Trial 61 finished with value: 16.485512457870527 and parameters: {'beta': 0.038013386697241885, 'init_prev': 0.03698830506986972, 'n_contacts': 6, 'rand_seed': 451598}. Best is trial 24 with value: 7.4876053262421465.
[I 2024-11-18 15:46:05,271] Trial 62 finished with value: 20.645747977225938 and parameters: {'beta': 0.03724420122914017, 'init_prev': 0.03329418737601248, 'n_contacts': 9, 'rand_seed': 998886}. Best is trial 24 with value: 7.4876053262421465.
[I 2024-11-18 15:46:05,917] Trial 63 finished with value: 9.605646441582167 and parameters: {'beta': 0.03416660355860217, 'init_prev': 0.033198001099660084, 'n_contacts': 9, 'rand_seed': 883171}. Best is trial 24 with value: 7.4876053262421465.
[I 2024-11-18 15:46:06,138] Trial 64 finished with value: 7.521087647927516 and parameters: {'beta': 0.03396894448904844, 'init_prev': 0.026926507308411386, 'n_contacts': 9, 'rand_seed': 888778}. Best is trial 24 with value: 7.4876053262421465.
[I 2024-11-18 15:46:06,864] Trial 65 finished with value: 121.41509371603001 and parameters: {'beta': 0.04925640676797698, 'init_prev': 0.031211942959032433, 'n_contacts': 10, 'rand_seed': 821766}. Best is trial 24 with value: 7.4876053262421465.
[I 2024-11-18 15:46:06,920] Trial 66 finished with value: 85.8053154897832 and parameters: {'beta': 0.04568024491119891, 'init_prev': 0.027214750978154154, 'n_contacts': 10, 'rand_seed': 193617}. Best is trial 24 with value: 7.4876053262421465.
[I 2024-11-18 15:46:07,651] Trial 68 finished with value: 94.08938156313411 and parameters: {'beta': 0.019480281261066285, 'init_prev': 0.024427857560705826, 'n_contacts': 8, 'rand_seed': 952012}. Best is trial 24 with value: 7.4876053262421465.
[I 2024-11-18 15:46:07,728] Trial 67 finished with value: 102.93924826470607 and parameters: {'beta': 0.22608905083239123, 'init_prev': 0.024028213274682155, 'n_contacts': 8, 'rand_seed': 770243}. Best is trial 24 with value: 7.4876053262421465.
[I 2024-11-18 15:46:08,412] Trial 69 finished with value: 126.53311109037782 and parameters: {'beta': 0.059717905932776365, 'init_prev': 0.02679364401368573, 'n_contacts': 9, 'rand_seed': 884741}. Best is trial 24 with value: 7.4876053262421465.
[I 2024-11-18 15:46:08,712] Trial 70 finished with value: 144.06858487997283 and parameters: {'beta': 0.06072714289448748, 'init_prev': 0.026342873632708538, 'n_contacts': 9, 'rand_seed': 877863}. Best is trial 24 with value: 7.4876053262421465.
[I 2024-11-18 15:46:09,352] Trial 71 finished with value: 45.91340754517728 and parameters: {'beta': 0.021336749301573588, 'init_prev': 0.022466616038583197, 'n_contacts': 9, 'rand_seed': 843723}. Best is trial 24 with value: 7.4876053262421465.
[I 2024-11-18 15:46:09,665] Trial 72 finished with value: 7.422085139257206 and parameters: {'beta': 0.03129768774449097, 'init_prev': 0.029207868668660285, 'n_contacts': 9, 'rand_seed': 938411}. Best is trial 72 with value: 7.422085139257206.
[I 2024-11-18 15:46:10,430] Trial 73 finished with value: 7.440442816706081 and parameters: {'beta': 0.03089432037237172, 'init_prev': 0.02917387747474371, 'n_contacts': 9, 'rand_seed': 947143}. Best is trial 72 with value: 7.422085139257206.
[I 2024-11-18 15:46:10,644] Trial 74 finished with value: 29.726828830302793 and parameters: {'beta': 0.042334794278848384, 'init_prev': 0.029560907129236542, 'n_contacts': 9, 'rand_seed': 970758}. Best is trial 72 with value: 7.422085139257206.
[I 2024-11-18 15:46:11,098] Trial 75 finished with value: 11.443667004724148 and parameters: {'beta': 0.029414933512879018, 'init_prev': 0.029293568556321693, 'n_contacts': 10, 'rand_seed': 948116}. Best is trial 72 with value: 7.422085139257206.
[I 2024-11-18 15:46:11,245] Trial 76 finished with value: 141.31771588050174 and parameters: {'beta': 0.29919457350194373, 'init_prev': 0.02850677756195807, 'n_contacts': 10, 'rand_seed': 929181}. Best is trial 72 with value: 7.422085139257206.
[I 2024-11-18 15:46:11,557] Trial 77 finished with value: 126.39404352808629 and parameters: {'beta': 0.025442359502867194, 'init_prev': 0.02787648649721561, 'n_contacts': 5, 'rand_seed': 918372}. Best is trial 72 with value: 7.422085139257206.
[I 2024-11-18 15:46:11,778] Trial 78 finished with value: 20.154515043898527 and parameters: {'beta': 0.025174807669208654, 'init_prev': 0.0314138608497185, 'n_contacts': 9, 'rand_seed': 811229}. Best is trial 72 with value: 7.422085139257206.
[I 2024-11-18 15:46:12,072] Trial 79 finished with value: 9.130978180887496 and parameters: {'beta': 0.03441024205856876, 'init_prev': 0.03141404187980859, 'n_contacts': 9, 'rand_seed': 822136}. Best is trial 72 with value: 7.422085139257206.
[I 2024-11-18 15:46:12,212] Trial 80 finished with value: 173.21230650618668 and parameters: {'beta': 0.017273378742237996, 'init_prev': 0.025482462846777933, 'n_contacts': 2, 'rand_seed': 868716}. Best is trial 72 with value: 7.422085139257206.
[I 2024-11-18 15:46:12,522] Trial 81 finished with value: 173.18039566778566 and parameters: {'beta': 0.017196648995799466, 'init_prev': 0.04970552338377038, 'n_contacts': 2, 'rand_seed': 849470}. Best is trial 72 with value: 7.422085139257206.
[I 2024-11-18 15:46:12,717] Trial 82 finished with value: 8.285562945506854 and parameters: {'beta': 0.03580761410924173, 'init_prev': 0.03406578394454271, 'n_contacts': 8, 'rand_seed': 901672}. Best is trial 72 with value: 7.422085139257206.
[I 2024-11-18 15:46:13,050] Trial 83 finished with value: 8.445578462057483 and parameters: {'beta': 0.03283859515494461, 'init_prev': 0.034436007541312076, 'n_contacts': 9, 'rand_seed': 909944}. Best is trial 72 with value: 7.422085139257206.
[I 2024-11-18 15:46:13,239] Trial 84 finished with value: 7.358566073477732 and parameters: {'beta': 0.03263543670972483, 'init_prev': 0.02780055255994975, 'n_contacts': 9, 'rand_seed': 711727}. Best is trial 84 with value: 7.358566073477732.
[I 2024-11-18 15:46:13,579] Trial 85 finished with value: 18.68796022610036 and parameters: {'beta': 0.028553575250512317, 'init_prev': 0.03004074412350957, 'n_contacts': 8, 'rand_seed': 707109}. Best is trial 84 with value: 7.358566073477732.
[I 2024-11-18 15:46:13,775] Trial 86 finished with value: 9.481302216551512 and parameters: {'beta': 0.027912689229722502, 'init_prev': 0.027708388127133318, 'n_contacts': 10, 'rand_seed': 703759}. Best is trial 84 with value: 7.358566073477732.
[I 2024-11-18 15:46:14,101] Trial 87 finished with value: 69.85886956915942 and parameters: {'beta': 0.04125061543778793, 'init_prev': 0.027994735538881897, 'n_contacts': 10, 'rand_seed': 962531}. Best is trial 84 with value: 7.358566073477732.
[I 2024-11-18 15:46:14,298] Trial 88 finished with value: 54.08499070148787 and parameters: {'beta': 0.04897060562863037, 'init_prev': 0.026206291751240778, 'n_contacts': 9, 'rand_seed': 765801}. Best is trial 84 with value: 7.358566073477732.
[I 2024-11-18 15:46:14,591] Trial 89 finished with value: 151.58323895691024 and parameters: {'beta': 0.10346189571740862, 'init_prev': 0.024866155083350706, 'n_contacts': 9, 'rand_seed': 759853}. Best is trial 84 with value: 7.358566073477732.
[I 2024-11-18 15:46:14,804] Trial 90 finished with value: 47.1334692289081 and parameters: {'beta': 0.02108253058694573, 'init_prev': 0.024709845478120513, 'n_contacts': 9, 'rand_seed': 946037}. Best is trial 84 with value: 7.358566073477732.
[I 2024-11-18 15:46:15,095] Trial 91 finished with value: 47.63757887948191 and parameters: {'beta': 0.020906565893169447, 'init_prev': 0.022670366440144106, 'n_contacts': 9, 'rand_seed': 988558}. Best is trial 84 with value: 7.358566073477732.
[I 2024-11-18 15:46:15,309] Trial 92 finished with value: 15.785253828324812 and parameters: {'beta': 0.03012472842769676, 'init_prev': 0.029089569588251558, 'n_contacts': 8, 'rand_seed': 609599}. Best is trial 84 with value: 7.358566073477732.
[I 2024-11-18 15:46:15,605] Trial 93 finished with value: 11.303565466922691 and parameters: {'beta': 0.0314644225778602, 'init_prev': 0.030607555378636297, 'n_contacts': 8, 'rand_seed': 484636}. Best is trial 84 with value: 7.358566073477732.
[I 2024-11-18 15:46:15,818] Trial 94 finished with value: 12.69544032126646 and parameters: {'beta': 0.03590738826199686, 'init_prev': 0.030778704663682405, 'n_contacts': 9, 'rand_seed': 483350}. Best is trial 84 with value: 7.358566073477732.
[I 2024-11-18 15:46:16,153] Trial 95 finished with value: 11.09579066483525 and parameters: {'beta': 0.03504729293003088, 'init_prev': 0.032080897860852865, 'n_contacts': 9, 'rand_seed': 587010}. Best is trial 84 with value: 7.358566073477732.
[I 2024-11-18 15:46:16,438] Trial 96 finished with value: 22.269822069457177 and parameters: {'beta': 0.023335449477639197, 'init_prev': 0.032051053297160806, 'n_contacts': 9, 'rand_seed': 861276}. Best is trial 84 with value: 7.358566073477732.
[I 2024-11-18 15:46:16,710] Trial 97 finished with value: 22.269822069457177 and parameters: {'beta': 0.023366025333188562, 'init_prev': 0.033581592959768675, 'n_contacts': 9, 'rand_seed': 646139}. Best is trial 84 with value: 7.358566073477732.
[I 2024-11-18 15:46:16,870] Trial 98 finished with value: 13.349747815915862 and parameters: {'beta': 0.06867747142655588, 'init_prev': 0.02728205632088007, 'n_contacts': 4, 'rand_seed': 894923}. Best is trial 84 with value: 7.358566073477732.
[I 2024-11-18 15:46:17,199] Trial 99 finished with value: 90.4451878578327 and parameters: {'beta': 0.04350581139573961, 'init_prev': 0.03997743638450222, 'n_contacts': 10, 'rand_seed': 531649}. Best is trial 84 with value: 7.358566073477732.
Making results structure...
Processed 100 trials; 0 failed
Best pars: {'beta': 0.03263543670972483, 'init_prev': 0.02780055255994975, 'n_contacts': 9, 'rand_seed': 711727}
Removed existing calibration file starsim_calibration.db
Let’s look at the best parameters that were found. Note that the rand_seed
was selected at random, but the other parameters are meaningful.
[7]:
calib.best_pars
[7]:
{'beta': 0.03263543670972483,
'init_prev': 0.02780055255994975,
'n_contacts': 9,
'rand_seed': 711727}
Once the calibration is complete, we can compare the guess
values to the best values found by calling check_fit
.
[8]:
# Confirm
sc.printcyan('\nConfirming fit...')
calib.check_fit(n_runs=5)
Confirming fit...
Checking fit...
Elapsed time: 1.57 s
Elapsed time: 1.77 s
Fit with original pars: [102.88081432 103.17668444 101.76293553 101.73024849 101.42137967]
Fit with best-fit pars: [ 9.24892243 8.48817549 8.04413658 11.3944745 10.23133013]
✓ Calibration improved fit
[8]:
(array([102.88081432, 103.17668444, 101.76293553, 101.73024849,
101.42137967]),
array([ 9.24892243, 8.48817549, 8.04413658, 11.3944745 , 10.23133013]))
Finally, we can view some plots of the results. Blue is before calibration using the guess
values whereas orange is after.
[9]:
calib.plot_sims()
[9]:
(<Figure size 933.333x700 with 12 Axes>,
<Figure size 933.333x700 with 12 Axes>)
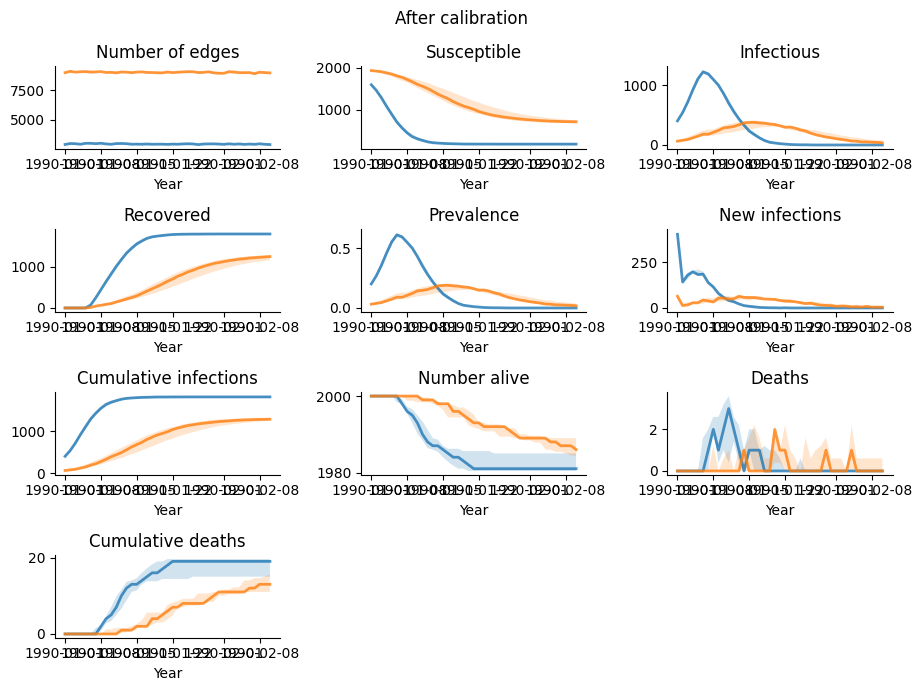
[10]:
calib.plot_trend()
[10]:
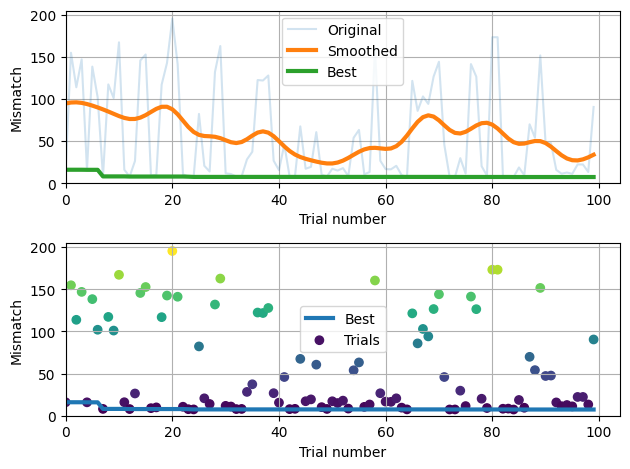