T1 - Getting started#
Installing and getting started with Covasim is quite simple.
To install, just type pip install covasim
. If it worked, you should be able to import Covasim with import covasim as cv
.
The basic design philosophy of Covasim is: common tasks should be simple. For example:
Defining parameters
Running a simulation
Plotting results
This tutorial walks you through how to do these things.
Click here to open an interactive version of this notebook.
Hello world#
To create, run, and plot a sim with default options is just:
[1]:
import covasim as cv
sim = cv.Sim()
sim.run()
fig = sim.plot()
Covasim 3.1.6 (2024-01-28) — © 2020-2024 by IDM
Initializing sim with 20000 people for 60 days
Running 2020-03-01 ( 0/60) (0.12 s) ———————————————————— 2%
Running 2020-03-11 (10/60) (0.16 s) •••————————————————— 18%
Running 2020-03-21 (20/60) (0.20 s) ••••••—————————————— 34%
Running 2020-03-31 (30/60) (0.24 s) ••••••••••—————————— 51%
Running 2020-04-10 (40/60) (0.29 s) •••••••••••••——————— 67%
Running 2020-04-20 (50/60) (0.35 s) ••••••••••••••••———— 84%
Running 2020-04-30 (60/60) (0.52 s) •••••••••••••••••••• 100%
Simulation summary:
12,730 cumulative infections
596 cumulative reinfections
10,182 cumulative infectious
6,395 cumulative symptomatic cases
386 cumulative severe cases
114 cumulative critical cases
5,159 cumulative recoveries
14 cumulative deaths
0 cumulative tests
0 cumulative diagnoses
0 cumulative known deaths
0 cumulative quarantined people
0 cumulative isolated people
0 cumulative vaccine doses
0 cumulative vaccinated people
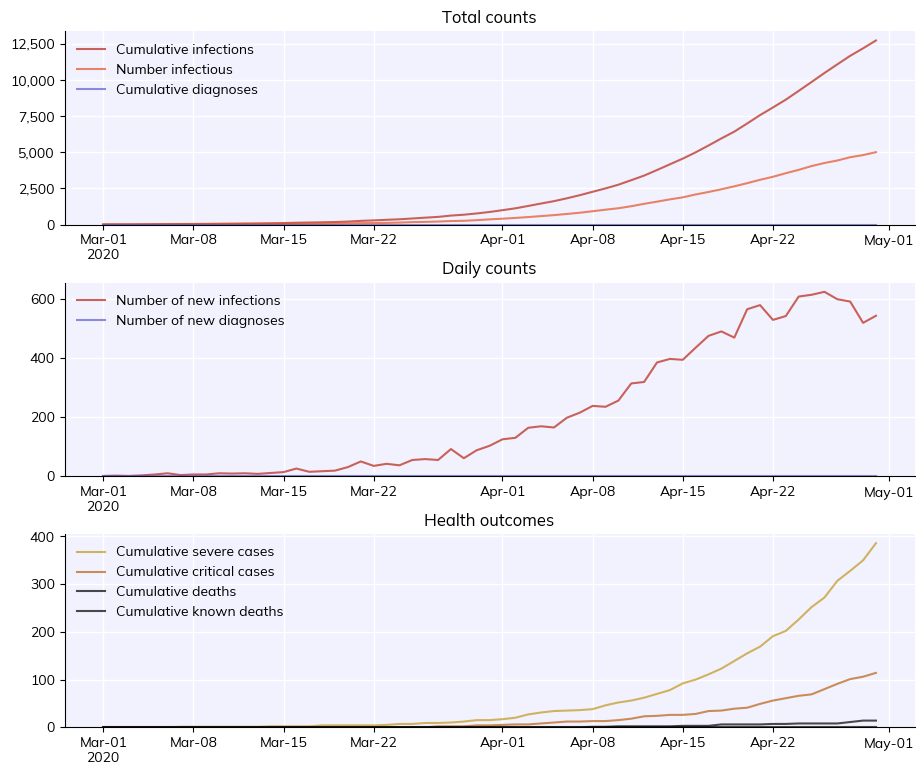
Defining parameters and running simulations#
Parameters are defined as a dictionary. The most common parameters to modify are the population size, the initial number of people infected, and the start and end dates of the simulation. We can define those as:
[2]:
pars = dict(
pop_size = 50e3,
pop_infected = 100,
start_day = '2020-04-01',
end_day = '2020-06-01',
)
Running a simulation is pretty easy. In fact, running a sim with the parameters we defined above is just:
[3]:
sim = cv.Sim(pars)
sim.run()
Initializing sim with 50000 people for 61 days
Running 2020-04-01 ( 0/61) (0.26 s) ———————————————————— 2%
Running 2020-04-11 (10/61) (0.34 s) •••————————————————— 18%
Running 2020-04-21 (20/61) (0.42 s) ••••••—————————————— 34%
Running 2020-05-01 (30/61) (0.52 s) ••••••••••—————————— 50%
Running 2020-05-11 (40/61) (0.64 s) •••••••••••••——————— 66%
Running 2020-05-21 (50/61) (0.80 s) ••••••••••••••••———— 82%
Running 2020-05-31 (60/61) (0.99 s) •••••••••••••••••••— 98%
Simulation summary:
41,466 cumulative infections
3,679 cumulative reinfections
36,997 cumulative infectious
24,043 cumulative symptomatic cases
1,542 cumulative severe cases
475 cumulative critical cases
24,320 cumulative recoveries
86 cumulative deaths
0 cumulative tests
0 cumulative diagnoses
0 cumulative known deaths
0 cumulative quarantined people
0 cumulative isolated people
0 cumulative vaccine doses
0 cumulative vaccinated people
[3]:
Sim(<no label>; 2020-04-01 to 2020-06-01; pop: 50000 random; epi: 41466⚙, 86☠)
This will generate a results dictionary sim.results
. For example, the number of new infections per day is sim.results['new_infections']
.
Rather than creating a parameter dictionary, any valid parameter can also be passed to the sim directly. For example, exactly equivalent to the above is:
[4]:
sim = cv.Sim(pop_size=50e3, pop_infected=100, start_day='2020-04-01', end_day='2020-06-01')
sim.run()
Initializing sim with 50000 people for 61 days
Running 2020-04-01 ( 0/61) (0.28 s) ———————————————————— 2%
Running 2020-04-11 (10/61) (0.36 s) •••————————————————— 18%
Running 2020-04-21 (20/61) (0.44 s) ••••••—————————————— 34%
Running 2020-05-01 (30/61) (0.54 s) ••••••••••—————————— 50%
Running 2020-05-11 (40/61) (0.66 s) •••••••••••••——————— 66%
Running 2020-05-21 (50/61) (0.82 s) ••••••••••••••••———— 82%
Running 2020-05-31 (60/61) (1.01 s) •••••••••••••••••••— 98%
Simulation summary:
41,466 cumulative infections
3,679 cumulative reinfections
36,997 cumulative infectious
24,043 cumulative symptomatic cases
1,542 cumulative severe cases
475 cumulative critical cases
24,320 cumulative recoveries
86 cumulative deaths
0 cumulative tests
0 cumulative diagnoses
0 cumulative known deaths
0 cumulative quarantined people
0 cumulative isolated people
0 cumulative vaccine doses
0 cumulative vaccinated people
[4]:
Sim(<no label>; 2020-04-01 to 2020-06-01; pop: 50000 random; epi: 41466⚙, 86☠)
You can mix and match too – pass in a parameter dictionary with default options, and then include other parameters as keywords (including overrides; keyword arguments take precedence). For example:
[5]:
sim = cv.Sim(pars, pop_infected=10) # Use parameters defined above, except start with 10 infected people
sim.run()
Initializing sim with 50000 people for 61 days
Running 2020-04-01 ( 0/61) (0.28 s) ———————————————————— 2%
Running 2020-04-11 (10/61) (0.34 s) •••————————————————— 18%
Running 2020-04-21 (20/61) (0.42 s) ••••••—————————————— 34%
Running 2020-05-01 (30/61) (0.50 s) ••••••••••—————————— 50%
Running 2020-05-11 (40/61) (0.58 s) •••••••••••••——————— 66%
Running 2020-05-21 (50/61) (0.67 s) ••••••••••••••••———— 82%
Running 2020-05-31 (60/61) (0.78 s) •••••••••••••••••••— 98%
Simulation summary:
10,392 cumulative infections
128 cumulative reinfections
6,710 cumulative infectious
4,185 cumulative symptomatic cases
195 cumulative severe cases
51 cumulative critical cases
2,473 cumulative recoveries
7 cumulative deaths
0 cumulative tests
0 cumulative diagnoses
0 cumulative known deaths
0 cumulative quarantined people
0 cumulative isolated people
0 cumulative vaccine doses
0 cumulative vaccinated people
[5]:
Sim(<no label>; 2020-04-01 to 2020-06-01; pop: 50000 random; epi: 10392⚙, 7☠)
Plotting results#
As you saw above, plotting the results of a simulation is rather easy too:
[6]:
fig = sim.plot()
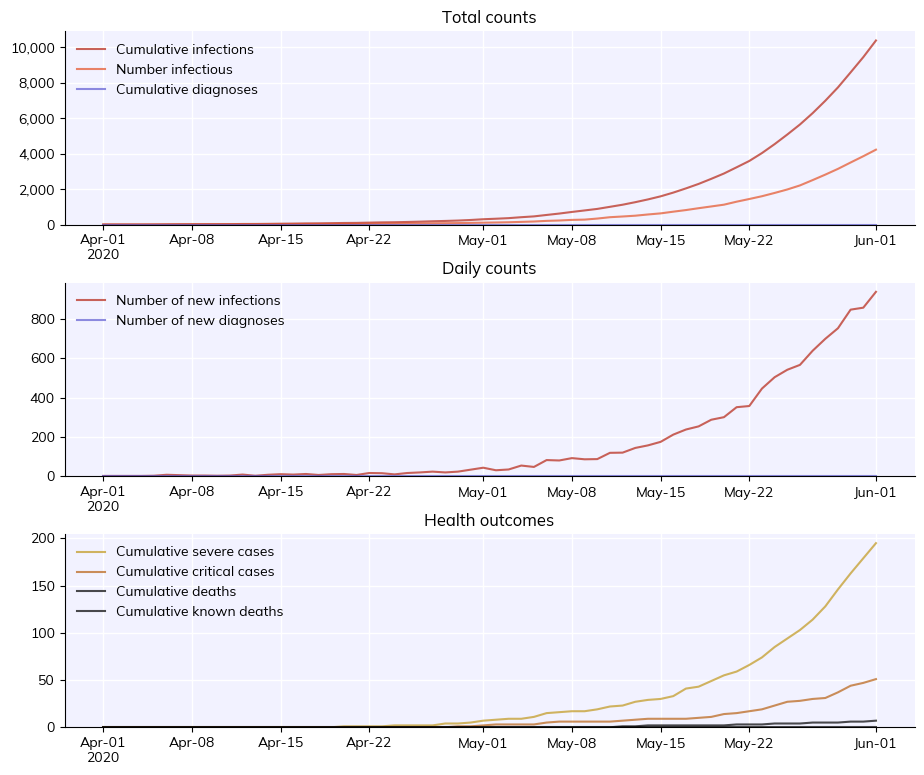
Full usage example#
Many of the details of this example will be explained in later tutorials, but to give you a taste, here’s an example of how you would run two simulations to determine the impact of a custom intervention aimed at protecting the elderly.
[7]:
import covasim as cv
# Custom intervention -- see Tutorial 5
def protect_elderly(sim):
if sim.t == sim.day('2020-04-01'):
elderly = sim.people.age>70
sim.people.rel_sus[elderly] = 0.0
pars = dict(
pop_type = 'hybrid', # Use a more realistic population model
location = 'japan', # Use population characteristics for Japan
pop_size = 50e3, # Have 50,000 people total in the population
pop_infected = 100, # Start with 100 infected people
n_days = 90, # Run the simulation for 90 days
verbose = 0, # Do not print any output
)
# Running with multisims -- see Tutorial 3
s1 = cv.Sim(pars, label='Default')
s2 = cv.Sim(pars, interventions=protect_elderly, label='Protect the elderly')
msim = cv.MultiSim([s1, s2])
msim.run()
fig = msim.plot(['cum_deaths', 'cum_infections'])
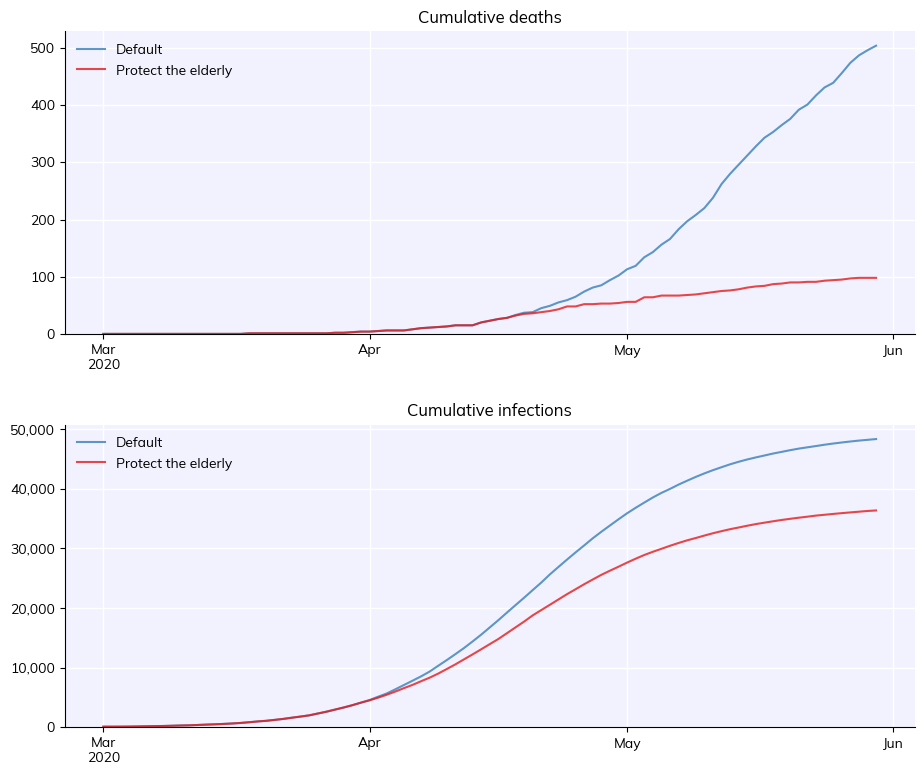