T10 - Tips and tricks#
This tutorial contains suggestions that aren’t essential to follow, but which may make your life easier.
Click here to open an interactive version of this notebook.
Getting help#
In addition to the docs (yes, you’re reading them as we speak, well done), you can get help within Covasim. You can even search for a string in Covasim’s source code. Curious to know where binomial distributions are used? You can do:
[1]:
import covasim as cv
cv.options(jupyter=True)
cv.help('binomial', source=True)
Covasim 3.1.6 (2024-01-28) — © 2020-2024 by IDM
Found 16 matches for "binomial" among 113 available functions:
People: 6 matches
binomial_arr: 3 matches
binomial_filter: 3 matches
contact_tracing: 1 matches
historical_vaccinate_prob: 3 matches
historical_wave: 1 matches
make_pars: 1 matches
make_random_contacts: 2 matches
make_randpop: 1 matches
n_binomial: 3 matches
n_neg_binomial: 5 matches
sample: 5 matches
simple_vaccine: 1 matches
test_prob: 1 matches
vaccinate_num: 1 matches
vaccinate_prob: 1 matches
You can also get a detailed view of the options with cv.options.help(detailed=True)
, or more concise ones with cv.options.help()
or cv.options.disp()
:
[2]:
cv.options.disp()
Covasim options (see also cv.options.help()):
verbose: 0.1
style: 'covasim'
dpi: 100
font: ['sans-serif']
fontsize: 10
interactive: True
jupyter: True
show: 1
close: 0
returnfig: False
backend: 'module://matplotlib_inline.backend_inline'
rc: {'axes.axisbelow': True,
'axes.spines.right': False,
'axes.spines.top': False,
'figure.facecolor': 'white',
'font.family': 'Mulish',
'legend.frameon': False,
'axes.facecolor': '#f2f2ff',
'axes.grid': True,
'grid.color': 'white',
'grid.linewidth': 1}
warnings: 'warn'
sep: ','
precision: 32
numba_parallel: 'none'
numba_cache: True
Versioning#
Covasim contains a number of built-in tools to make it easier to keep track of where results came from. The simplest of these is that if you save an image using cv.savefig()
instead of pl.savefig()
, it will automatically store information about the script and Covasim version that generated it:
[3]:
import covasim as cv
cv.options(jupyter=True, verbose=0)
sim = cv.Sim()
sim.run()
sim.plot()
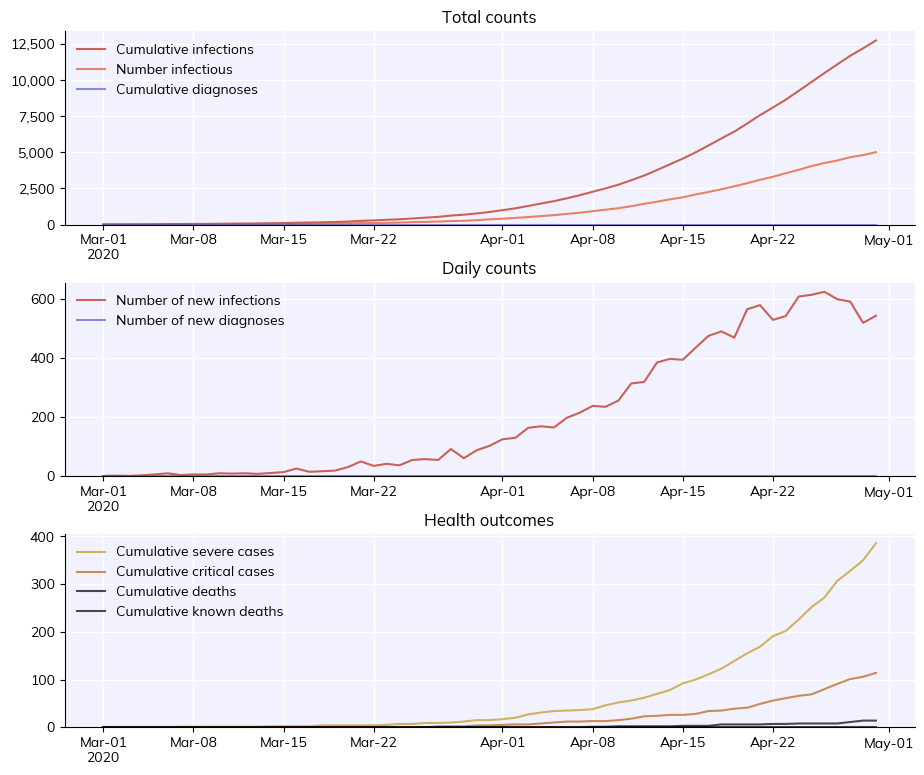
[4]:
filename = 'my-figure.png'
cv.savefig(filename) # Save including version information
cv.get_png_metadata(filename) # Retrieve and print information
Covasim version: 3.1.6
Covasim branch: Branch N/A
Covasim hash: Hash N/A
Covasim date: Date N/A
Covasim caller branch: Branch N/A
Covasim caller hash: Hash N/A
Covasim caller date: Date N/A
Covasim caller filename: /home/docs/checkouts/readthedocs.org/user_builds/institute-for-disease-modeling-covasim/envs/latest/lib/python3.9/site-packages/covasim/misc.py
Covasim current time: 2024-Mar-15 03:46:23
Covasim calling file: /tmp/ipykernel_2962/2013902684.py
This can be extremely useful for figuring out where that intriguing result you generated 3 weeks ago came from!
This information is also stored in sims and multisims themselves:
[5]:
print(sim.version)
print(sim.git_info)
3.1.6
{'covasim': {'version': '3.1.6', 'branch': 'Branch N/A', 'hash': 'Hash N/A', 'date': 'Date N/A'}, 'called_by': {'branch': 'Branch N/A', 'hash': 'Hash N/A', 'date': 'Date N/A', 'filename': '/tmp/ipykernel_2962/3079231718.py'}}
Finally, the function cv.check_version()
and cv.check_save_version()
are useful if you want to ensure that users are running the right version of your code. Placing cv.check_save_version('2.0.0')
will save a file with the information above to the current folder – again, useful for debugging exactly what changed and when. (You can also provide additional information to it, e.g. to also save the versions of 3rd-party packages you’re importing). cv.check_version()
by itself can be
used to provide a warning or even raise an exception (if die=True
) if the version is not what’s expected:
[6]:
cv.check_version('1.5.0')
Note: Covasim is newer than expected (3.1.6 vs. 1.5.0)
[6]:
1
Working with dates#
Dates can be tricky to work with. Covasim comes with a number of built-in features to work with dates. By default, by convention Covasim works with dates in the format YYYY-MM-DD
, e.g. '2020-12-01'
. However, it can handle a wide variety of other date and datetime
objects. In particular, sim
objects know when they start and end, and can use this to do quite a bit of date math:
[7]:
sim = cv.Sim(start_day='20201122', end_day='2020-12-09 02:14:58.727703')
sim.initialize() # Date conversion happens on initialization
print(sim['start_day'])
print(sim['end_day'])
print(sim.day(sim['end_day'])) # Prints the number of days until the end day, i.e. the length of the sim
2020-11-22
2020-12-09
17
You can also easily calculate the difference between two dates, or generate a range of dates. These are returned as strings by default, but can be converted to datetime objects via Sciris:
[8]:
import sciris as sc
print(cv.daydiff('2020-06-01', '2020-07-01', '2020-08-01'))
dates = cv.date_range('2020-04-04', '2020-04-12')
print(dates)
print(sc.readdate(dates))
[30, 31]
['2020-04-04', '2020-04-05', '2020-04-06', '2020-04-07', '2020-04-08', '2020-04-09', '2020-04-10', '2020-04-11', '2020-04-12']
[datetime.datetime(2020, 4, 4, 0, 0), datetime.datetime(2020, 4, 5, 0, 0), datetime.datetime(2020, 4, 6, 0, 0), datetime.datetime(2020, 4, 7, 0, 0), datetime.datetime(2020, 4, 8, 0, 0), datetime.datetime(2020, 4, 9, 0, 0), datetime.datetime(2020, 4, 10, 0, 0), datetime.datetime(2020, 4, 11, 0, 0), datetime.datetime(2020, 4, 12, 0, 0)]
Finally, one gotcha is that when loading Excel spreadsheets in pandas, dates are loaded in pandas’ internal Timestamp[ns64]
format, which nothing else seems to be able to read. If this happens to you, the solution (as far as Covasim is concerned) is to convert to a datetime.date
:
data = pd.read_excel(filename)
data['date'] = data['date'].dt.date
Working with dictionaries#
“I already know how to work with dictionaries”, you say. Yes, you do. But there are a couple tricks that might make things easier.
Covasim is built on Sciris, which includes containers odict
and objdict
. While these are documented elsewhere, a couple examples will serve to illustrate how they work.
An odict
is just an ordered dict that you can refer to by position as well as by key. For example:
[9]:
mydict = sc.odict(foo=[1,2,3], bar=[4,5,6]) # Assignment is the same as ordinary dictionaries
print('Entry foo:', mydict['foo'])
print('Entry 0:', mydict[0]) # Access by key or by index
for i,key,value in mydict.enumitems(): # Additional methods for iteration
print(f'Item {i} is named {key} and has value {value}')
Entry foo: [1, 2, 3]
Entry 0: [1, 2, 3]
Item 0 is named foo and has value [1, 2, 3]
Item 1 is named bar and has value [4, 5, 6]
An objdict
is exactly the same as an odict except it lets you reference keys as if they were attributes:
[10]:
myobjdict = sc.objdict(foo=[1,2,3], bar=[4,5,6])
print('Entry foo:', myobjdict['foo'])
print('Entry 0:', myobjdict[0]) # Access by key or by index
print('"Attribute" foo:', myobjdict.foo)
Entry foo: [1, 2, 3]
Entry 0: [1, 2, 3]
"Attribute" foo: [1, 2, 3]
Using this approach, you can get all the power and flexibility of dictionaries, while writing code as succinctly as possible. For example:
[11]:
total_pop = 44_483 # This many total people
pars= sc.objdict(
pop_type = 'hybrid',
pop_size = 10e3,
)
pars.pop_scale = total_pop/pars.pop_size # Instead of pars['pop_scale'] = total_pop/pars['pop_size']
sim = cv.Sim(**pars) # It's still a dict, so you can treat it as one!
For example, the results
object is an objdict
. This means that although you can use e.g. sim.results['new_infections']
, you can also use sim.results.new_infections
.
Finally, Sciris also contains a function called mergedicts
. This acts very similarly to dict.update()
, with the main difference being that it returns the result of merging the two dictionaries. This is especially useful for handling keyword arguments in functions:
[12]:
def myfunc(args=None, **kwargs):
defaults = dict(foo=[1,2,3], bar=[4,5,6])
merged_args = sc.mergedicts(defaults, args, kwargs)
print(merged_args)
myfunc(args=dict(bar=18), other_args='can be anything')
{'foo': [1, 2, 3], 'bar': 18, 'other_args': 'can be anything'}
As you can see, it merged the default settings, the arguments supplied to the function via the keyword args
, and then other keywords, into a single dictionary.