T6 - Using analyzers#
Analyzers are objects that do not change the behavior of a simulation, but just report on its internal state, almost always something to do with sim.people
. This tutorial takes you through some of the built-in analyzers and gives a brief example of how to build your own.
Click here to open an interactive version of this notebook.
Results by age#
By far the most common reason to use an analyzer is to report results by age. The results in sim.results
already include results disaggregated by age, e.g. sim.results['cancers_by_age']
, but these results use standardized age bins which may not match the age bins for available data on cervical cancers. Age-specific outputs can be customized using an analyzer to match the age bins of the data. The following example shows how to set this up:
[1]:
import numpy as np
import sciris as sc
import hpvsim as hpv
# Create some parameters, setting beta (per-contact transmission probability) higher
# to create more cancers for illutration
pars = dict(beta=0.5, n_agents=50e3, start=1970, n_years=50, dt=1., location='tanzania')
# Also set initial HPV prevalence to be high, again to generate more cancers
pars['init_hpv_prev'] = {
'age_brackets' : np.array([ 12, 17, 24, 34, 44, 64, 80, 150]),
'm' : np.array([ 0.0, 0.75, 0.9, 0.45, 0.1, 0.05, 0.005, 0]),
'f' : np.array([ 0.0, 0.75, 0.9, 0.45, 0.1, 0.05, 0.005, 0]),
}
# Create the age analyzers.
az1 = hpv.age_results(
result_args=sc.objdict(
hpv_prevalence=sc.objdict( # The keys of this dictionary are any results you want by age, and can be any key of sim.results
years=2019, # List the years that you want to generate results for
edges=np.array([0., 15., 20., 25., 30., 40., 45., 50., 55., 65., 100.]),
),
hpv_incidence=sc.objdict(
years=2019,
edges=np.array([0., 15., 20., 25., 30., 40., 45., 50., 55., 65., 100.]),
),
cancer_incidence=sc.objdict(
years=2019,
edges=np.array([0.,20.,25.,30.,40.,45.,50.,55.,65.,100.]),
),
cancer_mortality=sc.objdict(
years=2019,
edges=np.array([0., 20., 25., 30., 40., 45., 50., 55., 65., 100.]),
)
)
)
sim = hpv.Sim(pars, genotypes=[16, 18], analyzers=[az1])
sim.run()
a = sim.get_analyzer()
a.plot();
HPVsim 2.0.2 (2024-03-05) — © 2022-2024 by IDM
Loading location-specific demographic data for "tanzania"
Initializing sim with 50000 agents
Loading location-specific data for "tanzania"
Running 1970.0 ( 0/51) (1.11 s) ———————————————————— 2%
Running 1980.0 (10/51) (1.82 s) ••••———————————————— 22%
Running 1990.0 (20/51) (2.69 s) ••••••••———————————— 41%
Running 2000.0 (30/51) (3.79 s) ••••••••••••———————— 61%
Running 2010.0 (40/51) (5.26 s) ••••••••••••••••———— 80%
Running 2020.0 (50/51) (7.43 s) •••••••••••••••••••• 100%
Simulation summary:
7,080,056 total HPV infections
15,958 total cancers
13,253 total cancer deaths
1.03 mean HPV prevalence (%)
2.46 mean cancer incidence (per 100k)
64.72 mean age of infection (years)
76.25 mean age of cancer (years)
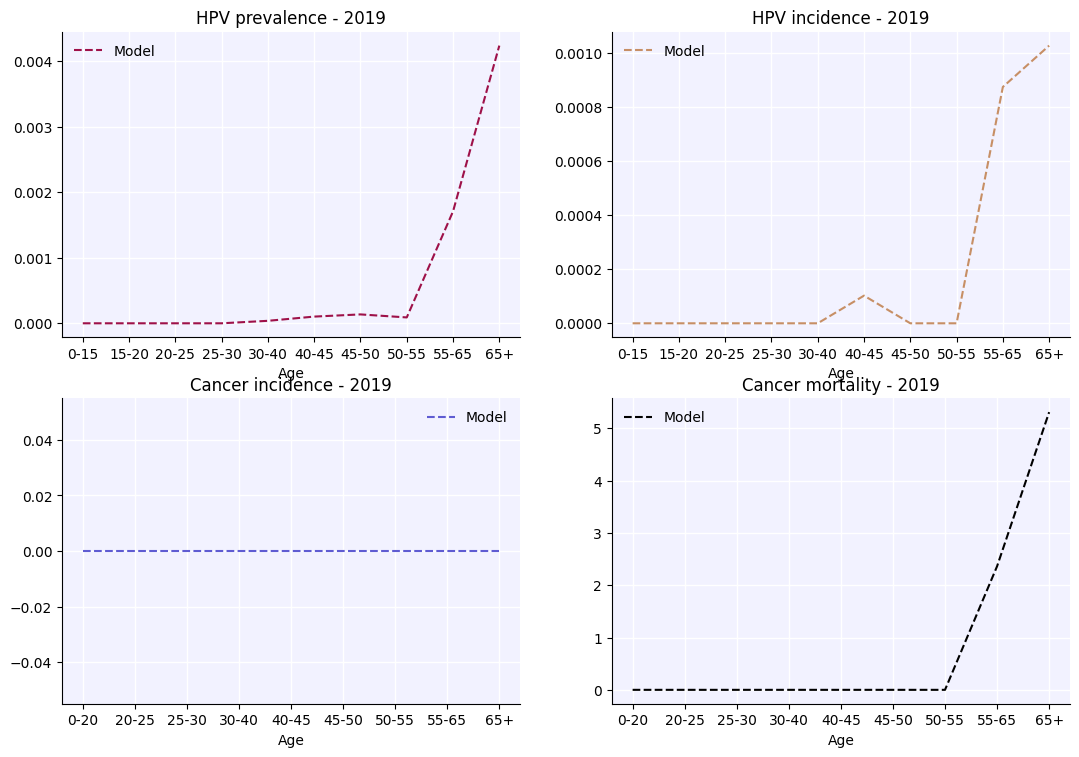
It’s also possible to plot these results alongside data.
[2]:
az2 = hpv.age_results(
result_args=sc.objdict(
cancers=sc.objdict(
datafile='example_cancer_cases.csv',
),
)
)
sim = hpv.Sim(pars, genotypes=[16, 18], analyzers=[az2])
sim.run()
a = sim.get_analyzer()
a.plot();
Loading location-specific demographic data for "tanzania"
Initializing sim with 50000 agents
Loading location-specific data for "tanzania"
Running 1970.0 ( 0/51) (0.09 s) ———————————————————— 2%
Running 1980.0 (10/51) (0.80 s) ••••———————————————— 22%
Running 1990.0 (20/51) (1.68 s) ••••••••———————————— 41%
Running 2000.0 (30/51) (2.78 s) ••••••••••••———————— 61%
Running 2010.0 (40/51) (4.24 s) ••••••••••••••••———— 80%
Running 2020.0 (50/51) (6.39 s) •••••••••••••••••••• 100%
Simulation summary:
7,080,056 total HPV infections
15,958 total cancers
13,253 total cancer deaths
1.03 mean HPV prevalence (%)
2.46 mean cancer incidence (per 100k)
64.72 mean age of infection (years)
76.25 mean age of cancer (years)
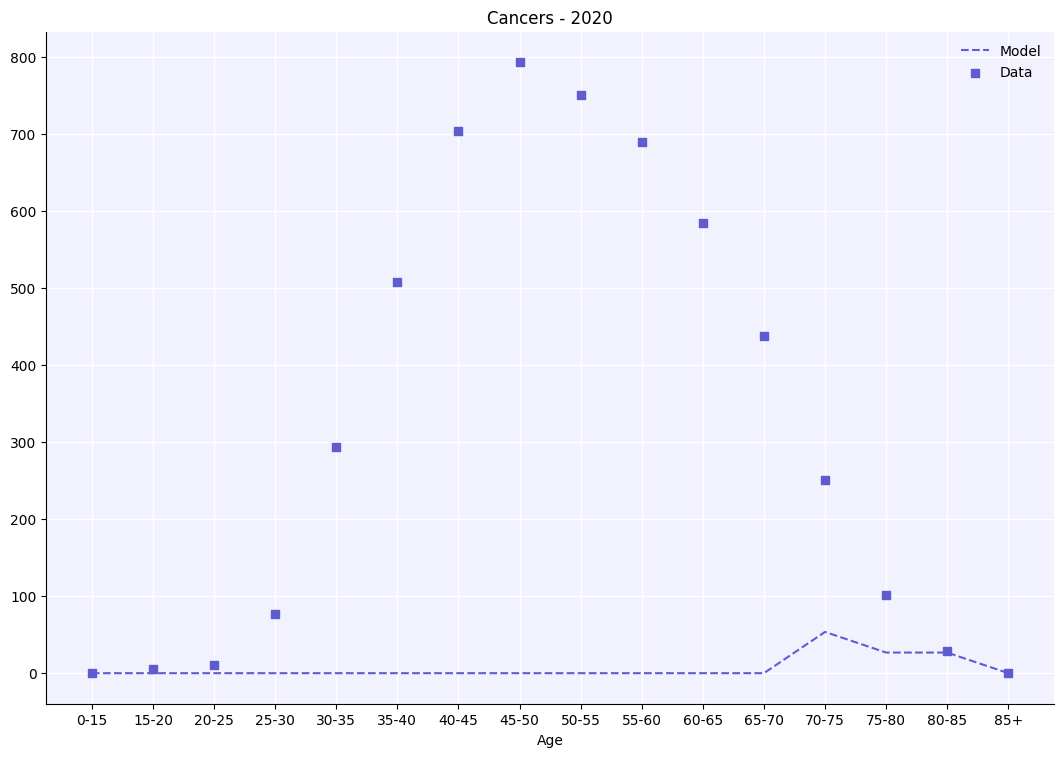
These results are not particularly well matched to the data, but we will deal with this in the calibration tutorial later.
Snapshots#
Snapshots both take “pictures” of the sim.people
object at specified points in time. This is because while most of the information from sim.people
is retrievable at the end of the sim from the stored events, it’s much easier to see what’s going on at the time. The following example leverages a snapshot in order to create a figure demonstrating age mixing patterns among sexual contacts:
[3]:
snap = hpv.snapshot(timepoints=['2020'])
sim = hpv.Sim(pars, analyzers=snap)
sim.run()
a = sim.get_analyzer()
people = a.snapshots[0]
# Plot age mixing
import pylab as pl
import matplotlib as mpl
fig, ax = pl.subplots(nrows=1, ncols=1, figsize=(5, 4))
fc = people.contacts['m']['age_f'] # Get the age of female contacts in marital partnership
mc = people.contacts['m']['age_m'] # Get the age of male contacts in marital partnership
h = ax.hist2d(fc, mc, bins=np.linspace(0, 75, 16), density=True, norm=mpl.colors.LogNorm())
ax.set_xlabel('Age of female partner')
ax.set_ylabel('Age of male partner')
fig.colorbar(h[3], ax=ax)
ax.set_title('Marital age mixing')
pl.show();
Loading location-specific demographic data for "tanzania"
Initializing sim with 50000 agents
Loading location-specific data for "tanzania"
Running 1970.0 ( 0/51) (0.11 s) ———————————————————— 2%
Running 1980.0 (10/51) (0.94 s) ••••———————————————— 22%
Running 1990.0 (20/51) (2.07 s) ••••••••———————————— 41%
Running 2000.0 (30/51) (3.86 s) ••••••••••••———————— 61%
Running 2010.0 (40/51) (6.07 s) ••••••••••••••••———— 80%
Running 2020.0 (50/51) (8.67 s) •••••••••••••••••••• 100%
Simulation summary:
7,169,617 total HPV infections
13,548 total cancers
10,978 total cancer deaths
0.67 mean HPV prevalence (%)
2.05 mean cancer incidence (per 100k)
67.17 mean age of infection (years)
74.17 mean age of cancer (years)
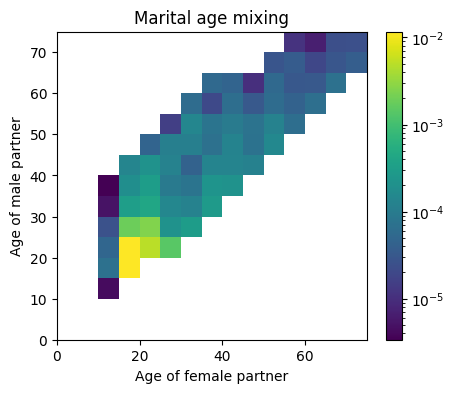
Age pyramids#
Age pyramids, like snapshots, take a picture of the people at a given point in time, and then bin them into age groups by sex. These can also be plotted alongside data:
[4]:
# Create some parameters
pars = dict(n_agents=50e3, start=2000, n_years=30, dt=0.5)
# Make the age pyramid analyzer
age_pyr = hpv.age_pyramid(
timepoints=['2010', '2020'],
datafile='south_africa_age_pyramid.csv',
edges=np.linspace(0, 100, 21))
# Make the sim, run, get the analyzer, and plot
sim = hpv.Sim(pars, location='south africa', analyzers=age_pyr)
sim.run()
a = sim.get_analyzer()
fig = a.plot(percentages=True);
Loading location-specific demographic data for "south africa"
Initializing sim with 50000 agents
Loading location-specific data for "south africa"
Dates provided in the age pyramid datafile ({'2020.0', '2000.0', '1990.0', '2010.0'}) are not the same as the age pyramid dates that were requested (['2010.0' '2020.0']).
Plots will only show requested dates, not all dates in the datafile.
Running 2000.0 ( 0/62) (0.10 s) ———————————————————— 2%
Running 2005.0 (10/62) (0.65 s) •••————————————————— 18%
Running 2010.0 (20/62) (1.19 s) ••••••—————————————— 34%
Running 2015.0 (30/62) (1.72 s) ••••••••••—————————— 50%
Running 2020.0 (40/62) (2.27 s) •••••••••••••——————— 66%
Running 2025.0 (50/62) (2.83 s) ••••••••••••••••———— 82%
Running 2030.0 (60/62) (3.41 s) •••••••••••••••••••— 98%
Simulation summary:
48,188,934 total HPV infections
46,514 total cancers
27,032 total cancer deaths
1.62 mean HPV prevalence (%)
5.21 mean cancer incidence (per 100k)
49.27 mean age of infection (years)
51.56 mean age of cancer (years)
/home/docs/checkouts/readthedocs.org/user_builds/institute-for-disease-modeling-hpvsim/envs/latest/lib/python3.9/site-packages/hpvsim/analysis.py:469: UserWarning: set_ticklabels() should only be used with a fixed number of ticks, i.e. after set_ticks() or using a FixedLocator.
ax.set_yticklabels(labels[1:])
/home/docs/checkouts/readthedocs.org/user_builds/institute-for-disease-modeling-hpvsim/envs/latest/lib/python3.9/site-packages/hpvsim/analysis.py:496: UserWarning: set_ticklabels() should only be used with a fixed number of ticks, i.e. after set_ticks() or using a FixedLocator.
ax.set_yticklabels(labels[1:])
/home/docs/checkouts/readthedocs.org/user_builds/institute-for-disease-modeling-hpvsim/envs/latest/lib/python3.9/site-packages/hpvsim/analysis.py:469: UserWarning: set_ticklabels() should only be used with a fixed number of ticks, i.e. after set_ticks() or using a FixedLocator.
ax.set_yticklabels(labels[1:])
/home/docs/checkouts/readthedocs.org/user_builds/institute-for-disease-modeling-hpvsim/envs/latest/lib/python3.9/site-packages/hpvsim/analysis.py:496: UserWarning: set_ticklabels() should only be used with a fixed number of ticks, i.e. after set_ticks() or using a FixedLocator.
ax.set_yticklabels(labels[1:])
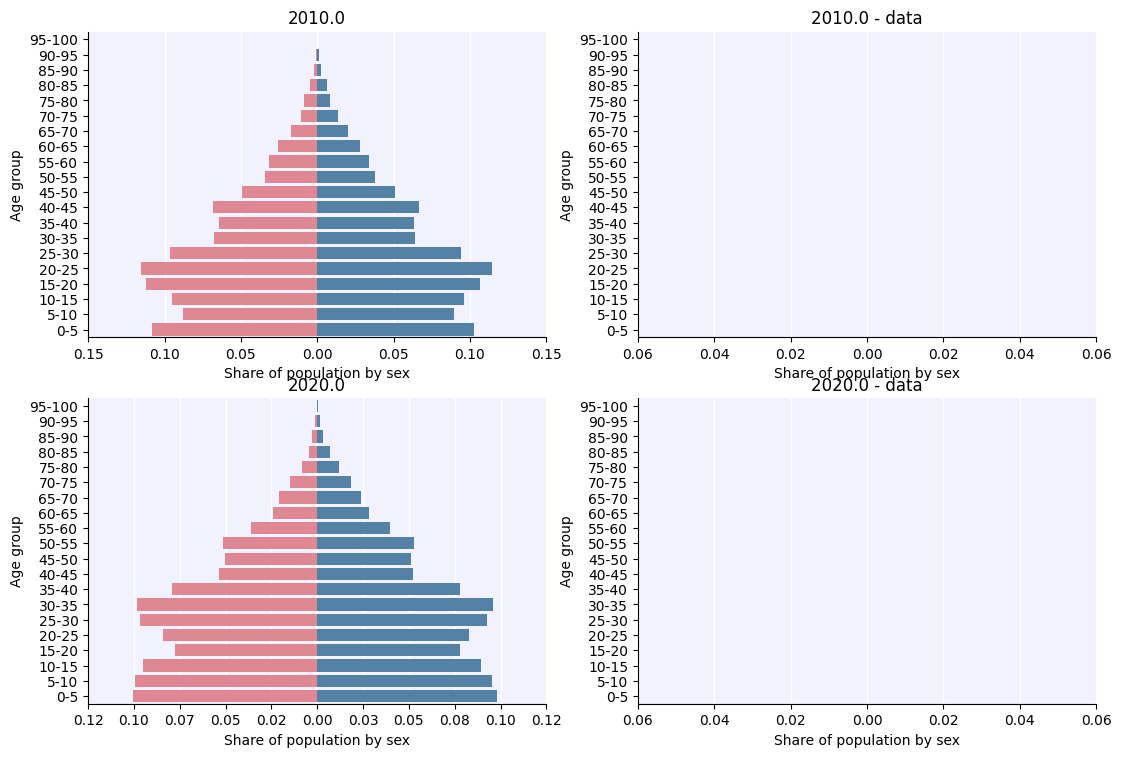